
There would be an equilibrium in the data flow when our capability of deciphering genomic data be enhanced. Hence, with efficient and cheaper NGS technologies, there is a great demand for efficient and cheaper data analysis tools as well. The other mentioned packages are expensive. Also, it leads to filling up of drives which can be an issue in itself, especially if you are doing a lot of analysis. Due to this disk writing activity is high and slows down the system. Please note that you can import evidence files with the read alignments into the CLC Genomics Workbench as well (refer to the Complete Genomics import section of the Workbench user. One serious drawback with Galaxy is that it stores results at every intermediate step. This is the file format used by Complete Genomics for all kinds of variant data and can be used to analyze and visualize the variant calls made by Complete Genomics.
#Clc genomics workbench invisible install#
Researchers can use the online version, install a local version on their server system or run Galaxy on the Amazon EC2 Cloud. Workflows are stored directly in a dedicated database, and jobs can be distributed onto a high-performance computing infrastructure. It contains scripts for over a 100 analysis tools and users can add new tools (requiring basic informatics skills) and share all analysis steps and pipelines. A CLC Genomics Workbench SNP report must include the following columns to be used. Galaxy is one of the most popular of analysis tools. SNP reports (CLC, Geneious, or VCF) must each correspond to a single.
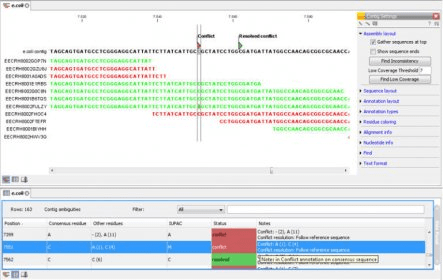
Few analysis packages include Galaxy, the CLC Genomics Workbench, DNANexus, and GenomeQuest. The major domains of NGS data analysis include genome annotation and gene prediction, differential expression analysis, Strutural variations, protein, DNA interactions, metagenomics data analysis, micro RNA analysis, etc.

Today one of the major bottleneck in NGS technology is data analysis. However, my concern is how much sense do these data make? Do we yet have the technologies to analyze these data efficiently? How much capable are we in converting these raw data into useful information? This will serve as a major challenge in the genomics field. By 2025, up to 2 billion, human genomes could be sequenced with the help of Next Generation Sequencing methods. According to the article, amount of genomic data that will be produced in the next 10 years is expected to be on par with or surpass that generated by astronomers, YouTube, or Twitter. I came across this recent article in "genomeweb" about the ever increasing amount of genomic data.
